The Marketer's Guide to A/B Testing: Optimizing Your Campaigns for Success
A/B testing is a powerful tool often overlooked by marketers as one of the most cost-effective ways to grow organic traffic. In the right hands, A/B testing is a marketer's arsenal, providing a scientific approach to optimizing campaigns and improving performance.
MARKETING STRATEGIESSTARTUPSSEARCH ENGINE OPTIMIZATIONGROWTHMONTHLY KPISGROWTH HACKINGA/B TESTING
Bill Arnold
8/31/202410 min read
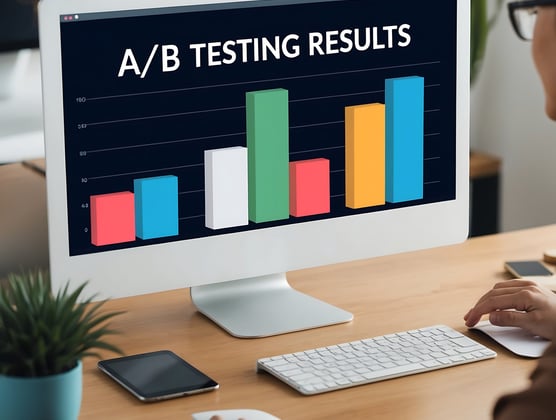
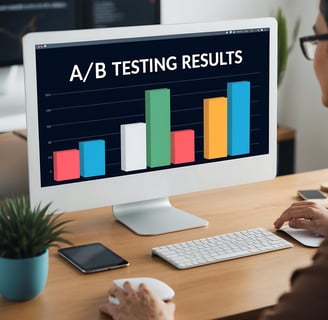
Introduction
A/B testing is a powerful tool often overlooked by marketers as one of the most cost-effective ways to grow organic traffic. In the right hands, A/B testing is a marketer's arsenal, providing a scientific approach to optimizing campaigns and improving performance.
By running controlled experiments, marketers can make data-driven decisions to enhance various aspects of their campaigns, from website copy and design to email subject lines and call-to-actions.
This blog will serve as an introduction for marketers seeking to understand and implement A/B testing protocols. It will focus on practical strategies and real-world examples. By the end, readers should feel equipped to design, execute, and analyze A/B tests that drive meaningful results for their organizations.
Understanding the Basics of A/B Testing
A/B testing, also known as split testing, compares two versions of a marketing asset to identify which performs better. It involves showing two variants (A and B) to similar audiences and measuring their impact on a predefined metric or key performance indicator (KPI).
The primary purpose of A/B testing is to make evidence-based decisions that improve campaign effectiveness. By testing different elements, marketers can identify the factors that resonate most with their target audience, leading to higher conversion rates and improved user experiences.
Common Use Cases
A/B testing is applicable across various marketing channels and assets. Here are some common use cases:
Website Optimization: Marketers can test different headlines, images, button colors, page layouts, and call-to-actions to increase conversions, whether it's for an e-commerce purchase, lead generation form submission, or newsletter sign-up.
Email Marketing: Subject lines, sender names, email body content, and design elements can be A/B tested to boost open rates, click-through rates, and conversions.
Landing Pages: Creating dedicated landing pages for specific campaigns and testing their elements can significantly impact the success of lead generation and sales efforts.
Advertising: A/B testing ad copy, visuals, and targeting options can help improve click-through rates and lower cost-per-acquisition in pay-per-click (PPC) campaigns.
Social Media: From organic posts to promoted content, social media marketers can test various elements to increase engagement, clicks, and conversions.
Influencer Marketing: Compare the effectiveness of influencers by comparing the five R's (Reach, Reputation, Resonance, Relevance, and Results) along with the cost to ascertain the best performing.
The opportunity for A/B testing is limited only by your imagination.
Key Terms and Concepts
Before diving into the practical aspects, it's essential to understand some key terms and concepts commonly used in A/B testing:
Variant: A variant is one of the versions of the marketing asset being tested. In a simple A/B test, there are two variants, A and B, each presented to a separate group of users.
Control and Treatment: The control is the original or current version (version A) against which the new variation (Version B) is tested. The treatment refers to the new version being tested, which is hypothesized to perform better.
Hypothesis: A hypothesis is a predicted outcome or expectation set before running the test. For example, "changing the button color from blue to green will increase click-throughs."
Statistical Significance: Statistical significance indicates that the results are unlikely due to chance and that the variation had a genuine impact. Common statistical significance levels are 90%, 95%, and 99%.
Confidence Interval: The confidence interval provides a range of values within which the true value of the metric being measured is likely to fall. A narrower interval indicates more precision.
Sample Size: The sample size refers to the number of participants or users included in the test. A sufficient sample size is crucial for achieving accurate and reliable results.
Conversion Rate: The conversion rate is the percentage of users who take the desired action (e.g., purchase, sign-up, click). It is often the primary metric used to determine the winning variation.
Implementing an A/B Testing Program
Step 1: Define Your Objectives and KPIs
Start by clearly defining the objectives of your A/B test. Why are you testing the particular variant and what is the anticipated outcome with the new variant.
Are you aiming to increase website sign-ups, improve email open rates, or boost sales through a specific call-to-action? Each objective will guide the design and execution of your test.
Once the objectives are set, identify the key performance indicators (KPIs) that align with those objectives. Examples of KPIs include click-through rates, conversion rates, average order value, revenue per visitor, and return on ad spend. Ensure your KPIs are specific, measurable, achievable, relevant, and time-bound (SMART).
Step 2: Identify Test Variables
Test variables are the elements of your marketing asset that you plan to change and evaluate. These could include headlines, images, body copy, call-to-actions, layout, color schemes, or form lengths. It's important to prioritize variables based on their potential impact on user behavior and your objectives.
For instance, if you aim to increase sign-ups for a free trial, you might hypothesize that changing the headline and reducing the number of form fields will lead to more conversions. In this case, the headline and form length would be your test variables.
Step 3: Determine Sample Size and Duration
Calculating the required sample size is crucial for ensuring accurate results. The sample size depends on several factors, including the amount of website traffic or email recipients, the baseline conversion rate, the minimum detectable effect, and the desired level of statistical confidence.
Numerous online calculators can help determine the required sample size for your test. As a rule of thumb, aim for a large enough sample to achieve statistical significance, typically above 95%.
The test duration will depend on the volume of traffic or interactions you receive. Tests can be shorter for websites with high traffic, while lower-traffic sites may require longer test durations.
PRO TIP: Be certain to allow enough time to obtain relevant and actionable data. The time required will depend on the variant you are testing and the traffic. Not allowing enough time can lead to misinterpreting the outcome.
Step 4: Choose an A/B Testing Tool
Several A/B testing tools are available, ranging from simple plugins to advanced platforms. Choose a tool that integrates well with your existing marketing stack and provides the necessary features for your specific use case.
For example, Google Optimize is a popular and free tool for website A/B testing, while email marketing platforms like HubSpot offer built-in A/B testing capabilities. More advanced tools like VWO (Visual Website Optimizer) and Optimizely provide additional features such as multivariate testing and personalized experiences.
Pro Tip: Prevail Marketing uses over 45 analytic tools specifically designed to measure a particular component. Do not try to save money by using a generic tool. Your analysis can only be as good as the data produced.
Step 5: Create Your Test Variations
Based on the test variables identified earlier, create the different variations of your marketing asset. For instance, if you're testing email subject lines, draft two or more subject lines incorporating different approaches or language. Ensure that only one variable is changed at a time to isolate the impact of each change. When creating variations, consider these best practices:
Keep it simple: Minor changes can often have a significant impact, so you don't need to overhaul your entire asset.
Challenge your assumptions: Test elements you may have initially dismissed to uncover unexpected insights.
Leverage personalization: Use dynamic content to personalize variations based on user data, such as names or purchase history.
Prioritize mobile: With the prevalence of mobile users, ensure your variations are optimized for mobile devices.
Pro Tip: Avoid the temptation to try multivariant testing, which involves testing more than two variables simultaneously. While you may improve the overall results quickly, you have no basis for knowing accurately what variant caused the improvement. This makes it challenging to apply the changes across your platform and even more difficult to know how to proceed with the next test.
Step 6: Set Up the Test
Use your chosen A/B testing tool to set up the experiment. This typically involves specifying the test duration, defining the audience, setting the allocation ratio, and determining the percentage of users who will see each variation.
Most tools will provide a visual editor or a code editor to make changes to your asset directly. Ensure that the variations are correctly implemented and that tracking codes are in place to capture the relevant data.
Step 7: Run the Test and Monitor Performance
Once the test is live, monitor its performance regularly, which means at least daily. While the test is running, avoid making changes to the test variables or the overall user experience, as this could impact the validity of your results.
Keep an eye on key metrics such as conversion rates, engagement, and user behavior. Some tools offer real-time reporting, allowing you to track the progress and spot any potential issues promptly.
Evaluating and Interpreting Test Results
Step 1: Analyze Statistical Significance
After the test duration has elapsed, it's time to analyze the results. Start by evaluating whether your test achieved statistical significance. This indicates that the difference in performance between the variations is likely due to the changes made and not random chance.
Most A/B testing tools will calculate statistical significance, typically represented as a p-value. A lower p-value indicates stronger evidence against the null hypothesis (no difference between the variations). A common threshold for statistical significance is a p-value of less than 0.05.
Step 2: Consider Practical Significance
Even if your test achieves statistical significance, it's important to consider practical significance. This refers to the magnitude of the difference between the variations and whether it has a meaningful impact on your business.
For example, a 0.2% uplift in conversion rate might be statistically significant but may not substantially impact your overall goals. Context matters, so consider the broader implications of the results and whether they align with your objectives.
Step 3: Evaluate Secondary Metrics
While your primary KPI is crucial, don't overlook secondary metrics. For instance, if you're testing a website's call-to-action, an increase in click-throughs is positive. Still, you should also consider the impact on downstream metrics such as purchase completion or customer satisfaction.
Evaluating secondary metrics provides a more holistic view of the test's impact and helps identify potential unintended consequences.
Step 4: Identify Patterns and Trends
Look for patterns and trends in the data that could provide additional insights. For example, you might discover that a specific variation performs better on mobile devices than desktops or that it resonates more with a particular demographic segment.
Understanding these patterns can help you further refine your marketing efforts and create more personalized experiences for different user segments.
Best Practices and Advanced Strategies for Successful A/B Testing
To maximize the success of your A/B testing program, consider the following best practices:
Start with a strong foundation: Ensure your website or landing page follows basic UX principles and best practices before running tests.
Prioritize tests: Focus on testing elements that have a higher potential impact on your goals. Prioritize based on expected impact and ease of implementation.
Isolate test variables: Only change one element at a time to attribute causality accurately. Otherwise, you won't know which change drove the observed effect.
Ensure sufficient traffic: Achieve a sample size that is large enough to ensure the validity of your results. Avoid making decisions based on tests with insufficient data.
Test above the fold: Prime real estate is the section of your website or email that users see without scrolling. Prioritize testing elements in this area for maximum visibility and impact.
Be patient: Allow the test to run its course, especially if you have lower traffic volumes. Prematurely ending a test could lead to inaccurate conclusions.
Continuous Improvement: Don't assume that you're done just because the B variant has improved the performance of what you were testing. Virtually everything can be improved.
Pro Tip: We had a client whose landing page had a conversion rate of 2.75%. At the end of the 30-day cycle we set aside for that initiative, we had increased the conversion rate to 19.76%. That only tells part of the story. The quality of the leads was dramatically better than what they had previously experienced. They experienced a 39% increase in leads who moved down the sales funnel to become customers.
Over those 30 days, the entire process involved over 15 separate A/B tests. We only stopped and moved on because we had identified other areas with a higher priority, meaning improvements in those areas would have a higher impact.
Advanced Strategies
As you become more proficient with A/B testing, consider these advanced strategies:
Personalization: Use A/B testing to optimize personalized experiences for different user segments. This could involve testing different offers, messages, or designs based on user demographics, behavior, or preferences.
Heatmap Analysis: Combine A/B testing with heatmap tools to visualize user behavior. Heatmaps can reveal how users interact with your variations, providing additional context to your test results.
Test Across Devices: Ensure your variations are optimized for different devices, including mobile, tablet, and desktop. Test the impact of changes on each device to cater to your audience's preferences.
Test Timing: Experiment with different timing for your marketing communications. For example, test sending emails at different times of the day or days of the week to identify the optimal send time.
Measuring Success and Optimizing Your Program
Defining Success Metrics
To measure the success of your A/B testing program, define key success metrics that align with your overall marketing goals. These could include:
Conversion Rate Uplift: Measure the average uplift in conversion rates across your A/B tests. Aim for a continuous improvement in this metric over time.
Revenue Impact: Assess the financial impact of your tests. Calculate the increase in revenue attributed to the winning variations, taking into account the additional sales or conversions.
Test Velocity: Evaluate the efficiency of your testing program by tracking the number of tests completed per month or quarter. Aim to increase this metric to accelerate learning and optimization.
Test Win Rate: Calculate the percentage of tests that result in a statistically significant uplift. A higher win rate indicates a more effective testing program.
Optimizing Your Testing Program
To optimize your A/B testing program and drive continuous improvement:
Institutionalize Testing: Embed A/B testing into your organization's culture. Encourage a data-driven mindset and ensure testing is a standard practice across marketing teams.
Automate and Scale: Leverage technology to automate and scale your testing efforts. Invest in tools for more efficient testing, such as those with machine learning capabilities.
Prioritize Testing Opportunities: Identify the areas with the highest potential for improvement and focus your testing efforts there. Prioritize based on impact and ease of implementation.
Learn from Losses: Treat failed tests as valuable learning opportunities. Analyze why a test didn't produce the expected results and use those insights to refine future tests.
Share Insights: Disseminate the learnings from successful tests across your organization. This can help inform and improve other marketing initiatives and strategies.
Growth Hacking
If you can get buy-in from your management team, consider implementing a growth hacking program. This involves the most aggressive form of A/B testing, where the entire organization is devoted to continual and optimal improvement.
Conclusion
A/B testing is a powerful tool for marketers seeking to optimize their campaigns and enhance the user experience. By following the guidelines, best practices, and strategies outlined in this guide, marketers can design, execute, and evaluate A/B tests effectively.
Remember, A/B testing is an ongoing process of experimentation and learning. Embrace a culture of continuous improvement, and you'll be well on your way to driving better campaign performance and achieving your marketing goals. Stay curious, test boldly, and let the data guide your path to success!
Contact:
prevailer@prevail.marketing
(424) 484-9955